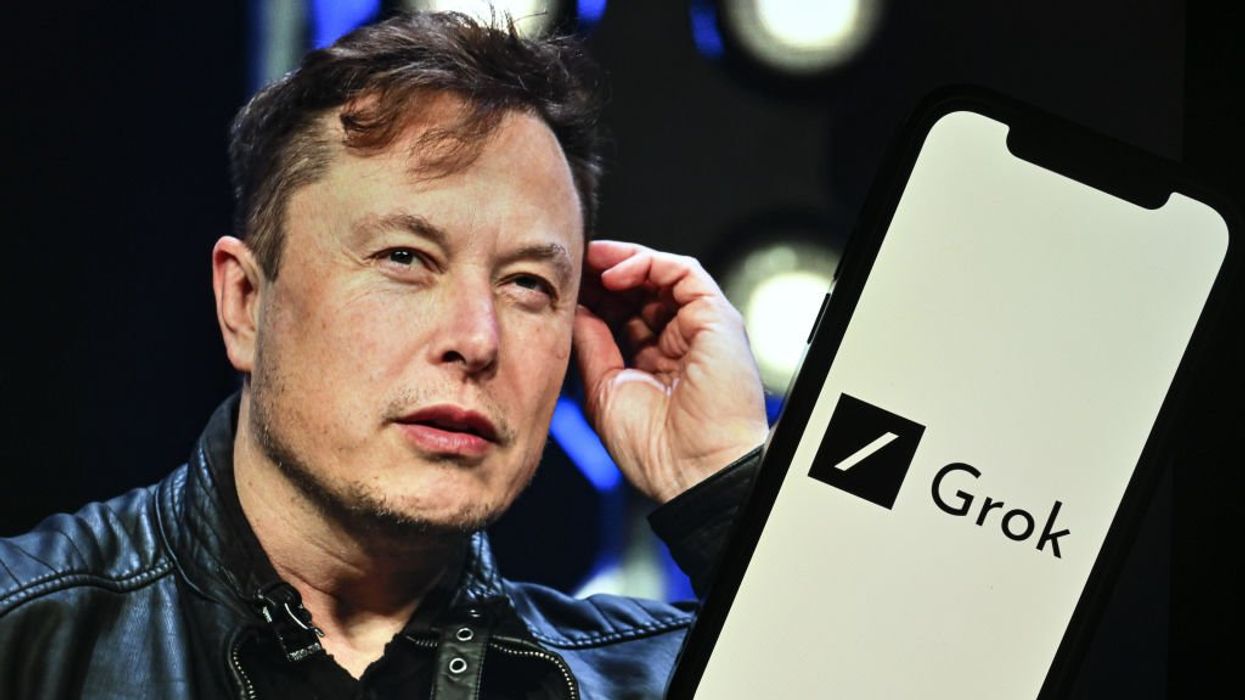
Anadolu/Getty
Large language models are transforming the very nature of information.
Elon Musk is one of the most polarizing figures on the planet — a part-time tech genius and full-time provocateur who never fails to get under the left's skin. His latest venture, xAI, has just unveiled a new image generation tool that is, as expected, stirring up inordinate amounts of controversy. This feature, designed to create a wide range of visuals, is accused of flooding the internet with deep fakes and other dubious imagery.
Among the content being shared are images of Donald Trump and a pregnant Kamala Harris as a couple and depictions of former presidents George W. Bush and Barack Obama with illegal substances. While these images have triggered the snowflake-like sensitivities of some on the left, those on the right might have more reason to be concerned about where this technology is headed. Let me explain.
This trend, coupled with the biases in training data, suggests that LLMs could continue to mirror and amplify left-leaning viewpoints.
To fully understand Grok's impact, it is crucial to see it within the broader AI landscape. Grok is a large language model, which places it among many others. The broader context reveals an important reality. The vast majority of LLMs tend to exhibit significant left-leaning biases.
LLMs are trained on vast amounts of internet data, which often skews toward progressive viewpoints. As a result, the outputs they generate can reflect these biases, influencing everything from political discourse to social media content.
A recent study by David Rozado, an AI researcher affiliated with Otago Polytechnic and Heterodox Academy, sheds light on a troubling trend in LLMs. Rozado analyzed 24 leading LLMs, including OpenAI’s GPT-3.5, GPT-4, Google’s Gemini, and Anthropic’s Claude, using 11 different political orientation evaluations. His findings reveal a consistent left-leaning bias across these models, with the “homogeneity of test results across LLMs developed by a wide variety of organizations is noteworthy” being particularly striking.
This situation becomes even more significant when considering the rapid evolution of search engines. As LLMs begin to replace traditional search engines, they are not just shifting our access to information; they are transforming it. Unlike search engines, which serve as vast digital libraries, LLMs are becoming personalized advisors, subtly curating the information we consume. This transition could make conventional search engines seem obsolete in comparison.
As Rozado points out, “The emergence of large language models (LLMs) as primary information providers marks a significant transformation in how individuals access and engage with information.” He adds, “Traditionally, people have relied on search engines or platforms like Wikipedia for quick and reliable access to a mix of factual and biased information. However, as LLMs become more advanced and accessible, they are starting to partially displace these conventional sources.”
Rozado further emphasizes, “This shift in the sourcing of information has profound societal implications, as LLMs can shape public opinion, influence voting behaviors, and impact the overall discourse in society. Therefore, it is crucial to critically examine and address the potential political biases embedded in LLMs to ensure a balanced, fair, and accurate representation of information in their responses to user queries.”
The study underscores the need to scrutinize the nature of bias in LLMs. Despite its obvious biases, traditional media allows for some degree of open debate and critique. In contrast, LLMs function in a far more opaque manner. They operate as black boxes, obscuring their internal processes and decision-making mechanisms. While traditional media can face challenges from a variety of angles, LLM content is more likely to escape such scrutiny.
Moreover, they don’t just retrieve information from the internet; they generate it based on the data they’ve been trained on, which inevitably reflects the biases present in that data. This can create an appearance of neutrality, hiding deeper biases that are more challenging to identify. For instance, if a specific LLM has a left-leaning bias, it might subtly favor certain viewpoints or sources over others when addressing sensitive topics like gender dysphoria or abortion. This can shape users' understanding of these issues not through explicit censorship but by subtly guiding content through algorithm-driven selection. Over time, this promotes a narrow range of perspectives while marginalizing others, effectively shifting the Overton window and narrowing the scope of acceptable discourse. Yes, things are bad now, but it’s difficult not to see them getting many times worse, especially if Kamala Harris, a darling of Silicon Valley, becomes president.
The potential implications of "LLM capture" are, for lack of a better word, severe. Given that many LLM developers come from predominantly left-leaning academic backgrounds, the biases from these environments may increasingly permeate the models themselves. This trend, coupled with the biases in training data, suggests that LLMs could continue to mirror and amplify left-leaning viewpoints.
Addressing these issues will require a concerted effort from respectable lawmakers (yes, a few of them still exist). Key to this will be improving transparency around the training processes of LLMs and understanding the nature of their biases. Jim Jordan and his colleagues recently had success dismantling GARM. Now, it’s time for them to turn their attention to a new, arguably far graver, threat.
John Mac Ghlionn
Contributor